Researchers at EPFL have developed a new, uniquely modular machine learning model for flexible decision-making. It is able to input any mode of text, video, image, sound, and time-series and then output any number, or combination, of predictions.
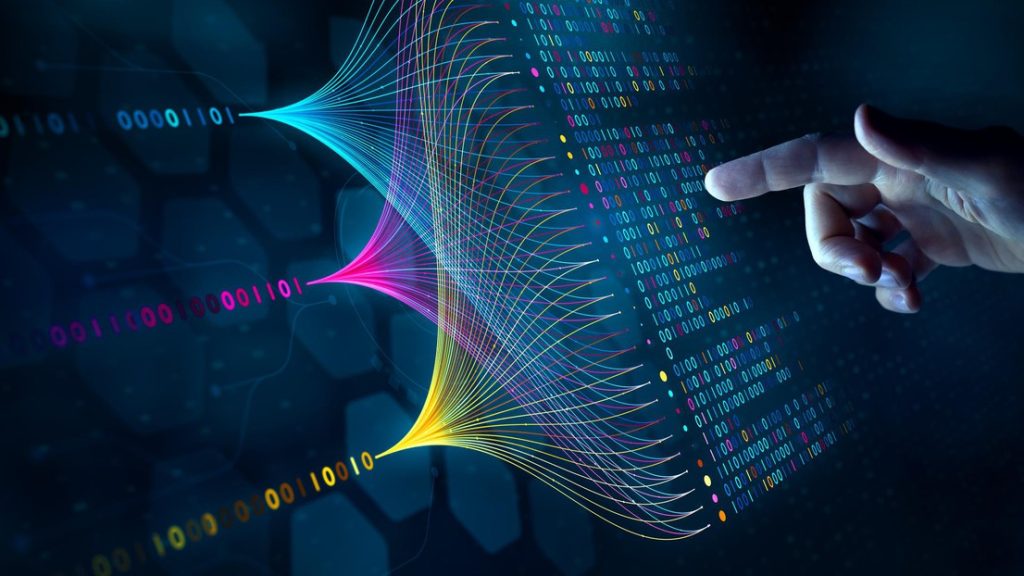
We’ve all heard of Large Language Models, or LLMs – massive scale deep learning models trained on huge amounts of text that form the basis for chatbots like OpenAI’s ChatGPT. Next-generation Multimodal Models (MMs) can learn from inputs beyond text, including video, images, and sound.
Creating MM models at a smaller scale poses significant challenges, including the problem of being robust to non-random missing information. This is information that a model doesn’t have, often due to some biased availability in resources. It is thus critical to ensure the model does not learn the patterns of biased missingness in making its predictions.
MultiModN turns this around
In response to this problem, researchers from the Machine Learning for Education (ML4ED) and Machine Learning and Optimization (MLO) Laboratories in EPFL’s School of Computer and Communication Sciences have developed and tested the exact opposite to a Large Language Model.
Spearheaded by Professor Mary-Anne Hartley, head of the Laboratory for intelligent Global Health Technologies hosted jointly in the MLO and the Yale School of Medicine and Professor Tanja Käser, head of ML4ED, MultiModN is a unique Modular Multimodal Model, presented recently at the NeurIPS2023 conference.
Like existing Multimodal Models MultiModN can learn from text, images, video, and sound. Unlike existing MMs, it is made up of any number of smaller, self-contained, and input-specific modules that can be selected depending on the information available, and then strung together in a sequence of any number, combination, or type of input. Itcan then output any number, or combination, of predictions.
“We evaluated MultiModN across ten real-world tasks including medical diagnosis support, academic performance prediction, and weather forecasting. Through these experiments, we believe that MultiModN is the first inherently interpretable, MNAR-resistant approach to multimodal modelling,” explained Vinitra Swamy, a PhD student with ML4ED and MLO and joint first author on the project.
A first use case: medical decision-making
The first use case for MultiModN will be as a clinical decision support system for medical personnel in low-resource settings. In healthcare, clinical data is often missing, perhaps due to resource constraints (a patient can’t afford the test) or resource abundance (the test is redundant due to a superior one that was performed). MultiModN is able to learn from this real-world data without adopting its biases, as well as adapting predictions to any combination or number of inputs.
“Missingness is a hallmark of data in low-resource settings and when models learn these patterns of missingness, they may encode bias into their predictions. The need for flexibility in the face of unpredictably available resources is what inspired MultiModN,” explained Hartley, who is also a medical doctor.
From the lab to real life
Publication, however, is just the first step towards implementation. Hartley has been working with colleagues at Lausanne University Hospital (CHUV) and Inselspital, University Hospital Bern uBern to conduct clinical studies focused on pneumonia and tuberculosis diagnosis in low resource settings and they are recruiting thousands of patients in South Africa, Tanzania, Namibia and Benin.
The research teams undertook a large training initiative, teaching more than 100 doctors to systematically collect multimodal data including images and ultrasound video, so that MultiModN can be trained to be sensitive to real data coming from low resource regions.
“We are collecting exactly the kind of complex multimodal data that MultiModN is designed to handle,” said Dr Noémie Boillat-Blanco, an infectious diseases doctor at CHUV. “We are excited to see a model that appreciates the complexity of missing resources in our settings and of systematic missingness of routine clinical assessments,” added Dr Kristina Keitel at Inselspital, University Hospital Bern.
Machine learning for the public good
The development and training of MultiModN is a continuation of EPFL efforts to adapt machine learning tools to reality and for the public good. It comes not long after the launch of Meditron, the world’s best performing open source LLM also designed to help guide clinical decision-making.
Dr. Mary-Anne Hartley is a keynote speaker at the upcoming Applied Machine Learning Days (AMLD) at the SwissTech Convention Center March 23-26, 2024. Vinitra Swamy will be presenting MultiModN at AMLD’s Applied eXplainable AI track. Discover other keynotes here and register here.
Author: Tanya Petersen