Sabine Süsstrunk, an expert in scientific photography, has seen first-hand the amazing progress in imaging technology over the past 40 years. And now her field is being upended by artificial intelligence (AI).
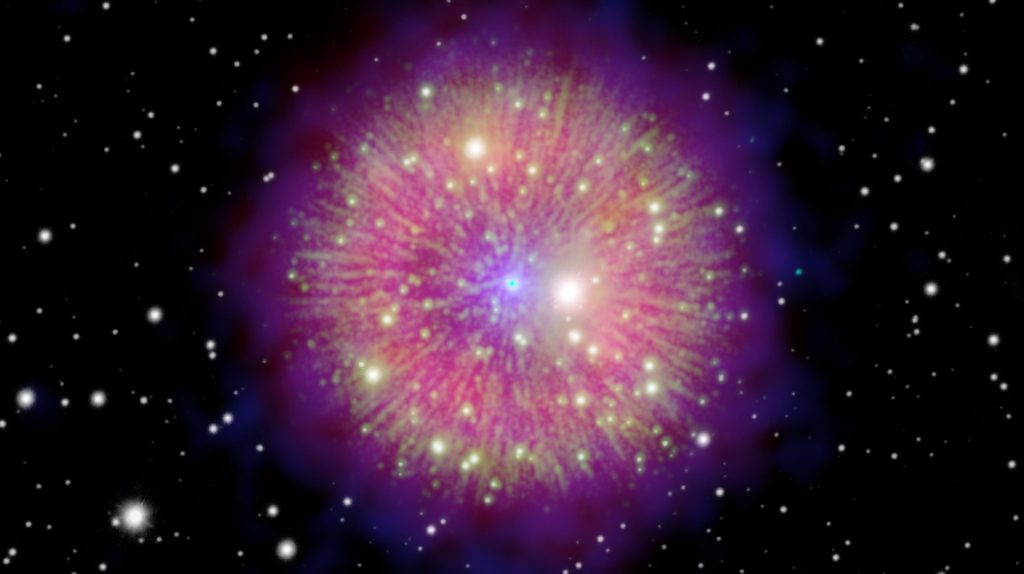
Prof. Süsstrunk is a computer scientist and has headed EPFL’s Images and Visual Representation Laboratory since 1999. She also serves on the Steering Committee for EPFL’s Center for Imaging and is the president of the Swiss Science Council.
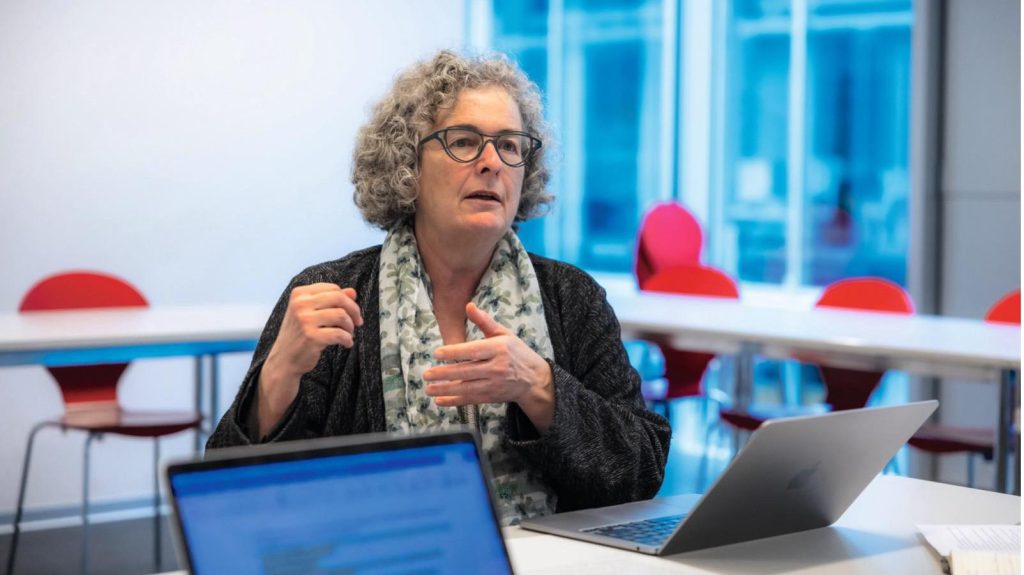
Sabine Süsstrunk – 2024 EPFL / Jamani Caillet – CC-BY-SA 4.0
Is there anything that can’t be seen today with imaging?
Everything in imaging is a matter of scale, from the infinite cosmos down to subatomic particles. Not to mention everyday imaging applications like materials science and medical diagnostics. Advances in imaging technology can occur along three dimensions: spatial, temporal or radiometric resolution. As instruments become more sensitive in these areas, they’ll be able to detect more things.
For example?
If we take remote sensing, devices are becoming increasingly powerful and capable of increasingly accurate measurements, such as to determine soil composition and moisture levels. This can help scientists better model the impact of growing certain crops, for example, and design more sustainable farming methods. Other goals with imaging are to detect cancer at an earlier stage, explore the interactions taking place within cells, unravel the mystery of the Big Bang and find life on exoplanets. In biology, recent advances in temporal resolution – instruments can now capture 1,000 or more images per second – are making it easier to decipher the movements and behavior of some animal species.
How is AI a game-changer for image generation?
In space observation, for example, as soon as a new telescope is installed we start learning new things. But it’s AI that enabled them to produce the first image of a black hole. It’s actually a virtuous circle: more advanced instruments can take better images for training AI programs, these programs then reconstruct images and help improve the instruments, thus resulting in better images for training AI programs, and so on. Imaging and AI go hand in hand. However, a vast number of images is required for AI, so we need instruments capable of generating images in large quantities.
And what about image analysis?
Well-trained AI programs are already better than humans at analyzing images. Breast cancer is detected more effectively by AI than by radiologists. The database of mammograms is so large that developers have been able to train AI programs extensively. But that’s also where the problem lies – AI is virtually incapable of detecting rare cancers or anything it hasn’t been trained on with enough datapoints. So in addition to a vast number of images, a wide variety is also important. The reliability of a system depends on the application and on how human researchers and AI have been trained. Neither is 100% reliable.
Could computer simulations one day replace imaging?
AI can overcome the limitations of physical imaging systems by reconstructing images so that they’re better than those taken by the system itself. Combining AI with super-resolution instruments, for example, can increase the spatial resolution by a factor of two, four or even eight. AI works by determining which piece of information is most likely to come next. If a program has been fed enough data, it can predict with a fairly high probability that a given data point will come after a pixel in an image that’s been captured by an instrument. That means AI can simulate things we aren’t able to see yet with physical imaging. But the catch is, we have no proof that these simulations are accurate.
Can AI get something completely wrong?
Absolutely. AI programs construct models that have a high probability of being correct – but that’s not the same as real-world data. AI-based images shouldn’t be used in studies where it’s important to observe real physical signals. Otherwise, that could lead to the same kinds of problems users run into with ChatGPT. AI operates according to how it’s been trained. If a program hasn’t been trained to recognize a given type of data or image, it won’t detect it. AI programs simply pick out what they’ve already seen. Another problem is that they’ll always generate an output – they’ll never simply say “I don’t know.” So the further you stray from the original application, the less reliable a program will be, whether for image generation, reconstruction or analysis.
“Scientists need to be transparent and ethical when using AI with imaging
– Sabine Süsstrunk
Could AI pose a threat to the quality and credibility of scientific publications?
Image generation technology clearly makes it easier to produce false images. But forgery was around well before AI. Research was conducted for a long time before the issues of open science and reproducibility arose. What’s important is the ethics of individual researchers.
How do you determine whether research results are reliable?
Personally, I’m doubtful when the authors don’t make their code available and don’t clearly indicate what kind of data were used. These are basic reproducibility criteria. I also don’t believe half of the research findings issued by large companies. That said, I do make an exception for medical studies, since the data are sensitive so they can’t be made public. But even when authors provide their code, it’s often hard to reproduce the results. You usually end up a few percentage points short.
Could researchers accidentally misuse AI with their images?
Imaging is becoming increasingly important to science in general as data analysis methods become cheaper and more powerful. But to use AI properly, scientists need to have a good understanding of imaging and know its limitations. Novices can easily be duped – but researchers have a responsibility to get the proper training. At EPFL’s Center for Imaging, we offer courses and summer programs for that reason.
Imaging lets scientists see the invisible at all levels, but can AI create things that don’t exist, like with deepfakes?
It all depends on how it’s used. Deepfakes in and of themselves aren’t a threat, but they could be if they’re used improperly, like if they go viral on social media. Photographs have long been manipulated to create images of scenes that don’t exist. But photographs are generally meant to be appealing – they just have to be visually plausible. Scientific images, on the other hand, aren’t meant to be aesthetic. They’re used to objectively measure or visualize a physical process, meaning they must be physically realistic. They’re intended to provide insight into what scientists are studying.
Does that mean only optical imaging can produce real images?
Yes, although even optical images can be compromised if there’s an error with the data acquisition. Another problem with optical images is that they contain noise, which has to be removed through reconstruction. If this isn’t done correctly, the images can be corrupted. But optical images are more reliable than those generated by AI.
Is processing power an obstacle?
It takes a long time to build new AI models, and you have to train algorithms with billions of parameters, which requires huge amounts of processing power. That’s why only heavyweights like OpenAI and Meta are doing it. But the foundation models they use can generate anything, including text, images, sound and video. In scientific research, however, we use single-task models designed for only one thing, such as to detect breast cancer or reconstruct MRI scans. These require much less processing power. The SwissAI initiative aims to develop such single-task models.
How can AI be improved?
One big problem is that the data for training AI programs have to be annotated. After you show three pictures of a cat to a one-year-old child, for example, she’ll be able to recognize a cat. But to train an algorithm, you need thousands of pictures that have been annotated by a human to indicate what’s shown in the image so that the program learns correctly. If you don’t annotate the data used to train an algorithm, it will be three times less accurate. Progress is really needed in this kind of self-supervised learning because, for now, we can’t use AI in applications for which we don’t have much data. This progress won’t happen right away, but it will happen.
What does the future hold?
If I could see the future, I would have already started my own company! Kidding aside, I think AI will help us develop better data acquisition technology. As I mentioned before, AI and imaging technology form a virtuous circle – better sensors result in better analysis, and better analysis leads to improved sensors, and so forth. This positive momentum will drive breakthroughs in all aspects of imaging, including spatial and temporal resolution. When imaging advances, science advances.
Authors: Anne-Muriel Brouet, Cécilia Carron
Source: EPFL