EPFL professor’s passion for sustainable flying and expertise in machine learning and computer vision drives innovation in green aviation design and beyond.
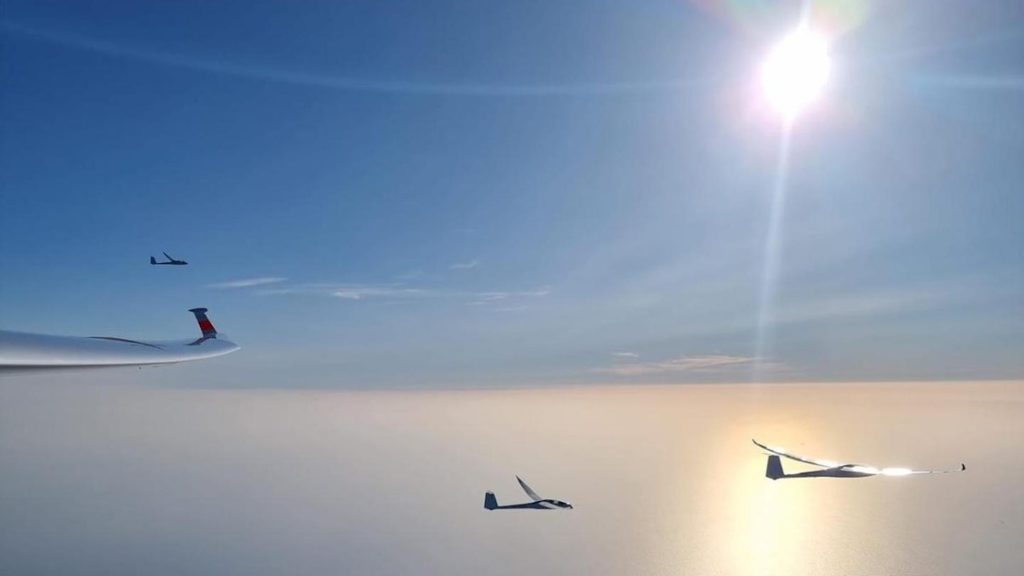
Aerodynamic shape optimization (ASO) is a key technique in aerodynamic design aimed at enhancing an object’s physical performance while adhering to specific constraints.
To optimize a 3D shape, one has to represent it in terms of a number of parameters that can be fed to the optimizer. A standard way to do this is to use a technique known as Free Form Deformations (FFD). Unfortunately, this requires extensive manual intervention and even when used by a domain expert, it often takes a great deal of trial and error to get right.
Deep Geometric Mapping
Now, researchers in the Computer Vision Laboratory (CVLab), part of the School of Computer and Communication Sciences(IC), in collaboration with colleagues at ISAE-SupAero in France, have developed the DeepGeo model, a fully automated neural-network-based approach to generating the required parameterization for complex geometries.
“DeepGeo performs the same function as FFD, but uses recent developments in deep learning to eliminate the need for human tinkering. It finds the appropriate parameters, which are then used to optimize the aircraft design. It is no longer necessary to spend months coming up with good parameterization,” explained Professor Pascal Fua, Head of the CV Lab.
DeepGeo also adapts the volumetric meshes modeling – the discretized computational domain surrounding the target object – as its shape changes during optimization. These volumetric meshes are necessary to perform the required Computational Fluid Dynamics computations and automating their deformation further reduces the workload of the designers.
Because it is innovative and potentially transformational, the corresponding paper, DeepGeo: Deep Geometric Mapping for Automated and Effective Parameterization in Aerodynamic Shape Optimization recently won a best student paper award at the biggest aeronautics conference of the year, the American Institute of Aeronautics and Astronautics Forum ‘24.
It outlines how DeepGeo is based on deep geometric learning techniques without requiring large-scale training datasets. Multiple case studies undertaken by the researchers, including 2D circle-to-airfoil optimization, 3D CRM wing optimization, and 3D Blended-Wing-Body aircraft optimization, demonstrate the effectiveness and robustness of DeepGeo. It delivers comparable performance to FFD at a fraction of the cost in terms of the required effort by human designers.
“By eliminating the need for extensive datasets and hyperparameter tuning, DeepGeo significantly reduces implementation complexity and cost and our research highlights its potential to automate ASO, making it more accessible and efficient,” added Zhen Wei, a doctoral assistant in the CV Lab and first author on the paper.
A personal passion driving green aviation design
For Fua, his drive to optimize green aviation design goes beyond the laboratory. He’s a passionate glider pilot and recently took part in a journey with motorized gliders from Chambéry in France to Ourzazate in Morocco.
Whilst the adventure was a lot of fun, Fua was constantly monitoring the sustainability of the trip and uses his amateur ‘in the air’ experience as inspiration for new research ideas. The expedition was soaring most of the time and only used the engines very sparingly. It covered more than 5000 km using the engine for less than 5 hours in a total of 55 hours flying time.
“For more sustainable aviation, the game is to essentially change the shape of aircraft to reduce drag. This is an old problem, it’s been around forever,” explained Fua. “Going forward we want to first use DeepGeo on model gliders used for FAI competitions. Because they need to fulfil so many contradictory requirements while being reasonably cheap to build, they are a wonderful test bed for our technology.”
Beyond sustainable aviation
Whilst model gliders will provide a demonstration of what DeepGeo can do, there’s a much bigger picture: This research is about optimizing design and efficiency for commercial jets and passenger airlines – planes with engines – and a wide range of applications beyond.
Beyond the field of aeronautics, the automated simultaneous design of shapes that interact, such as contiguous car components or internal parts of turbines, is a pervasive and open problem. One of Fua’s long term goals is to revolutionize the way that composite objects, whose parts can have arbitrary shapes or topologies, are modelled, manipulated and optimized, enforcing both design constraints on individual parts and compatibility constraints among these parts.
“DeepGeo offers a promising solution for complex geometric parameterization in the field of aerodynamic shape optimization. Future work in this direction will be key in designing energy-efficient machines at a time when minimizing humanity’s impact on the environment has become a critical concern,” Fua concluded.
Author: Tanya Petersen
Source: EPFL